A Unique Journey in Science and Technology
Stephen Wolfram, a renowned figure in the world of science and technology, embarked on an unconventional path that began with his early departure from both Eton and Oxford due to his insatiable curiosity. At the age of 20, he earned a doctorate in theoretical physics from Caltech, a testament to his exceptional intellect. He joined the faculty at Caltech in 1979, but his thirst for innovation and exploration led him to leave academia and create influential research tools like Mathematica, WolframAlpha, and the Wolfram Language. His book, ‘A New Kind of Science,’ a groundbreaking work that proposed that nature operates on ultrasimple computational rules, received both praise and criticism for its ambitious ideas.
Assessing the AI Panic
In a thought-provoking conversation with Reason’s Katherine Mangu-Ward, Wolfram delved into the varying reactions to AI’s rise. He highlighted the spectrum of opinions, from fears of AI dominance to skepticism about its capabilities. ‘I interact with lots of people,’ he shared, ‘and it ranges from those who are convinced that AIs are going to eat us all to those who say AIs are really stupid and won’t be able to do anything interesting.’ Wolfram’s perspective is one of optimism, as he sees technological advancements historically elevating human capabilities. AI, in his view, represents a significant step in this progression, a beacon of hope for the future.
Can AI Solve Science?
Wolfram delved into the thought-provoking question, ‘Can AI solve science?’ He explained that AI’s current approach, primarily through neural networks trained on human data, needs to improve with scientific predictions due to the concept of ‘computational irreducibility.’ This concept, he clarified, refers to the idea that in some systems, the only way to predict their behavior is to explicitly run the rules, making it a fundamental challenge in applying AI to natural phenomena. ‘to know what’s going to happen, you have to explicitly run the rules,’ he stated, highlighting the fundamental challenge in applying AI to natural phenomena. Unlike human-created language, which neural nets can predict, the physical world requires detailed computation to foresee outcomes.
The Changing Landscape of Academic Work
The introduction of AI-generated content has transformed academic work in various ways. Wolfram pointed out that the traditional effort associated with creating academic papers is diminishing. ‘If you write an academic paper, it’s just a bunch of words. Who knows whether there’s a brick there that people can build on?’ he questioned. He suggested that formalized knowledge, such as mathematics, offers a more solid foundation for future research compared to potentially unreliable AI-generated content. For instance, AI can now assist in data analysis, literature reviews, and even generate research questions, significantly reducing the time and effort required for these tasks.
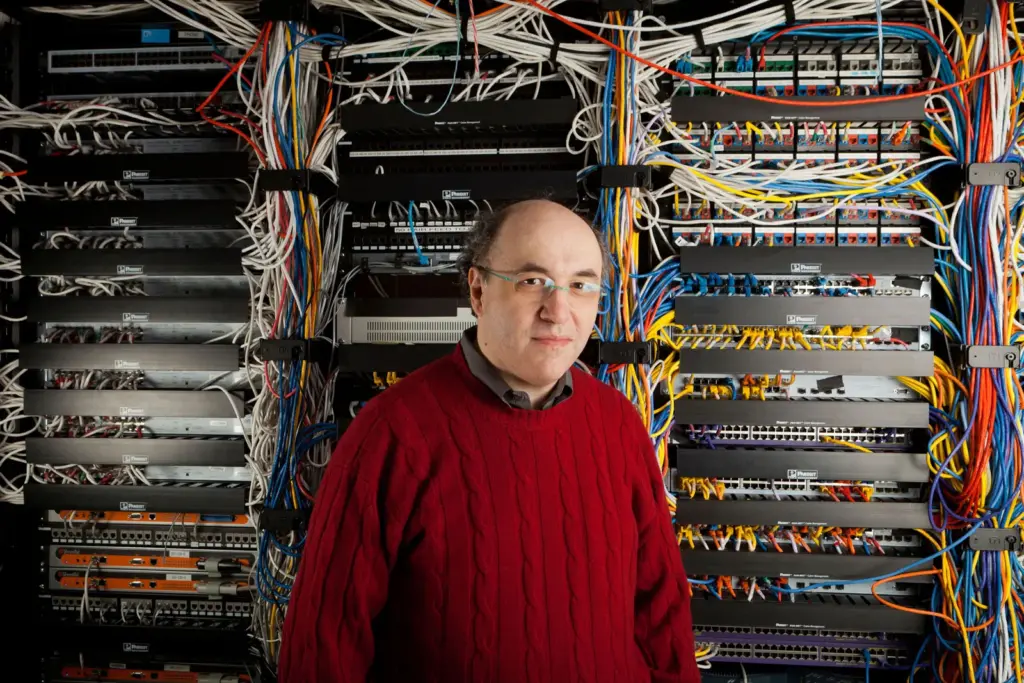
AI in Education and Tutoring
Wolfram’s team is developing an AI tutor capable of personalized instruction using large language models (LLMs). While initial demonstrations show promise, creating a robust system remains challenging. “The first things you try work for the two-minute demo and then fall over horribly,” Wolfram admitted. Nevertheless, AI’s ability to customize educational content to individual interests significantly advances human-computer interaction.
The Role of AI in Regulatory Processes
Wolfram highlighted AI’s potential to streamline regulatory processes. He envisioned a scenario where AI generates regulatory documents based on key points provided by humans, which are then reviewed by other AI systems. This could simplify complex bureaucratic interactions, though Wolfram expressed a preference for more direct communication with regulators. ‘Could we skip the elaborate regulatory filing, and they could just tell the five things directly to the regulators?’ he suggested. He also acknowledged the potential implications of AI in regulatory processes on transparency and accountability, such as the need to ensure that AI systems are unbiased and that the decision-making process is understandable and auditable.
The Need for New Political Philosophy
Reflecting on historical developments in political philosophy, Wolfram called for renewed thinking to address AI’s growing societal role. He proposed a “promptocracy,” where AI synthesizes citizen input to inform government decisions. “As we think about AIs that end up having responsibilities in the world, how do we deal with that?” he asked, emphasizing the need for innovative approaches to governance in the AI era.
Balancing AI Competition and Ethical Concerns
Wolfram discussed the importance of competition among AI systems to prevent monopolistic control and promote stability. He argued that multiple AI systems could mitigate the risk of extreme outcomes and foster innovation. ‘The society of AIs is more stable than the one AI that rules them all,’ he asserted. This competition, he suggested, is crucial for balancing ethical concerns and driving technological progress. However, he also acknowledged the potential impact of AI on the job market, such as the risk of job displacement or the need for new skills to adapt to the changing technological landscape.
Navigating the Risks and Rewards of AI
Addressing the potential risks and rewards of AI implementation, Wolfram cautioned that while AI could suggest actions with unpredictable consequences, it also holds the potential to revolutionize various industries and improve our daily lives. ‘AIs will be suggesting all kinds of things that one might do just as a GPS gives one directions for what one might do,’ he explained. Striking a balance between allowing AI autonomy and ensuring human oversight is essential to harnessing AI’s potential without stifling innovation. ‘The alternative is to tie it down to the point where it will only do the things we want it to do and it will only do things we can predict it will do. And that will mean it can’t do very much,’ he cautioned.
The Institutional Challenge in Science
Wolfram acknowledged the difficulty of fostering innovation within institutionalized science. While individual creativity is essential, collective efforts are necessary for significant breakthroughs. “Individual people can come up with original ideas. By the time it’s institutionalized, that’s much harder,” he noted. Despite this, he recognized the importance of large-scale collaborative efforts in advancing scientific knowledge.
Controversial Insights and Future Implications
Wolfram didn’t shy away from controversial statements during the conversation. He remarked on the limitations of current AI models, “Neural nets work well on things that we humans invented. They don’t work very well on things that are just sort of wheeled in from the outside world.” He also touched on the potential dangers of over-regulating AI, suggesting that excessive control could stifle innovation. “Be careful what you wish for,” he warned, “because you say, ‘I want lots of people to be doing this kind of science because it’s really cool and things can be discovered.’ But as soon as lots of people are doing it, it ends up getting this institutional structure that makes it hard for new things to happen.”
This interview has been condensed and edited for style and clarity.
Source: Reason
Like this article? Keep up to date with AI news, apps, tools and get tips and tricks on how to improve with AI. Sign up to our Free AI Newsletter
Also, come check out our free AI training portal and community of business owners, entrepreneurs, executives and creators. Level up your business with AI ! New courses added weekly.
You can also follow us on X